AI’s environmental impact is growing. Discover key strategies to reduce energy consumption with optimized models, AI chips, and renewable-powered data centers
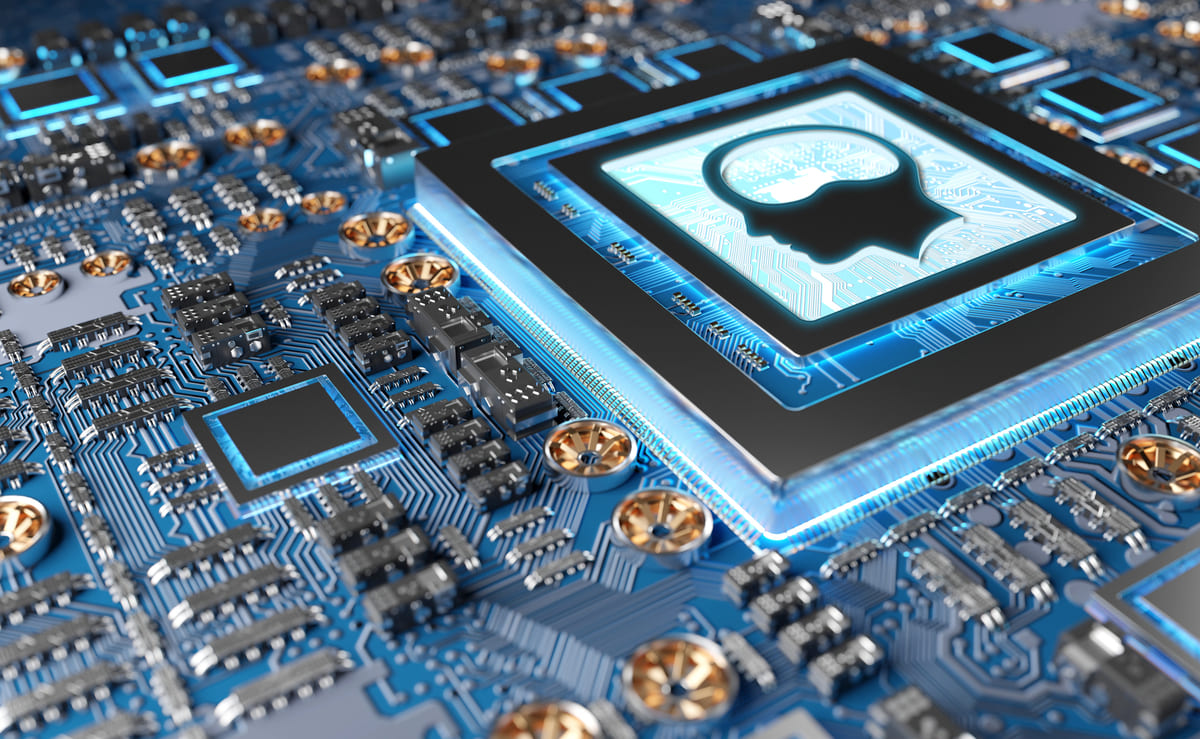
From pruning and carbon-aware scheduling to AI-optimized chips and increasing the share of renewables in the energy mix powering data centers—these are some of the key strategies to curb AI’s environmental footprint. A new report from Politecnico di Milano analyzes the energy consumption of AI models and suggests ways to mitigate it.
AI Energy Consumption: The Hidden Cost of Every Query
Asking a chatbot a question can consume up to 10 times more energy than a traditional web search (2.9 Wh vs. 0.3 Wh). Reducing this energy demand is crucial, as demonstrated by the recent case of DeepSeek, which was blocked in Italy due to privacy concerns. But how can we make AI greener?
While training AI models requires a significant amount of electricity, it’s not the biggest energy drain. Inference—the process of using a trained model to generate responses—accounts for 80-90% of AI’s total energy consumption. This happens because inference is performed millions of times a day, making it the most energy-intensive phase over the entire lifecycle of an AI model.
How to Reduce AI’s Environmental Impact?
The Digitalization & Decarbonization Report 2024, compiled by Politecnico di Milano’s Energy & Strategy group, explores AI’s energy footprint and identifies actionable strategies for reducing it.
Key Strategies to Lower AI’s Energy Demand
Algorithmic Optimization
- Pruning and quantization: Reducing model complexity without sacrificing performance.
- Knowledge distillation: Creating lightweight versions of AI models.
- Batch size and mixed-precision training: Enhancing efficiency in both training and inference.
Hardware Efficiency
- Using AI-optimized chips that require less energy per computation.
- Designing more efficient computing architectures to improve power consumption.
Operational Optimization
- Carbon-aware scheduling: Running AI workloads when renewable energy is most available.
- Green AI: Developing models with sustainability in mind.
Workload Distribution
- Leveraging federated learning and edge computing to reduce reliance on large, centralized data centers.
- Powering data centers with renewable energy to cut carbon emissions.
The Key Factors Driving AI’s Energy Consumption
According to Politecnico di Milano’s report, AI’s environmental impact depends on the energy sources powering data centers and the efficiency of both hardware and software. The report highlights six major factors influencing AI’s energy footprint:
Chip architecture – More advanced processors can improve computational efficiency and reduce power consumption.
Workload demand – The continuous use of AI in data centers creates significant energy needs.
Data center design – Infrastructure and layout impact overall energy efficiency.
Cooling systems – Inefficient cooling increases energy use.
Energy source – A higher share of renewables in the electricity mix can significantly cut CO₂ emissions.
Software optimization – Model compression and efficient inference can reduce AI’s environmental impact.