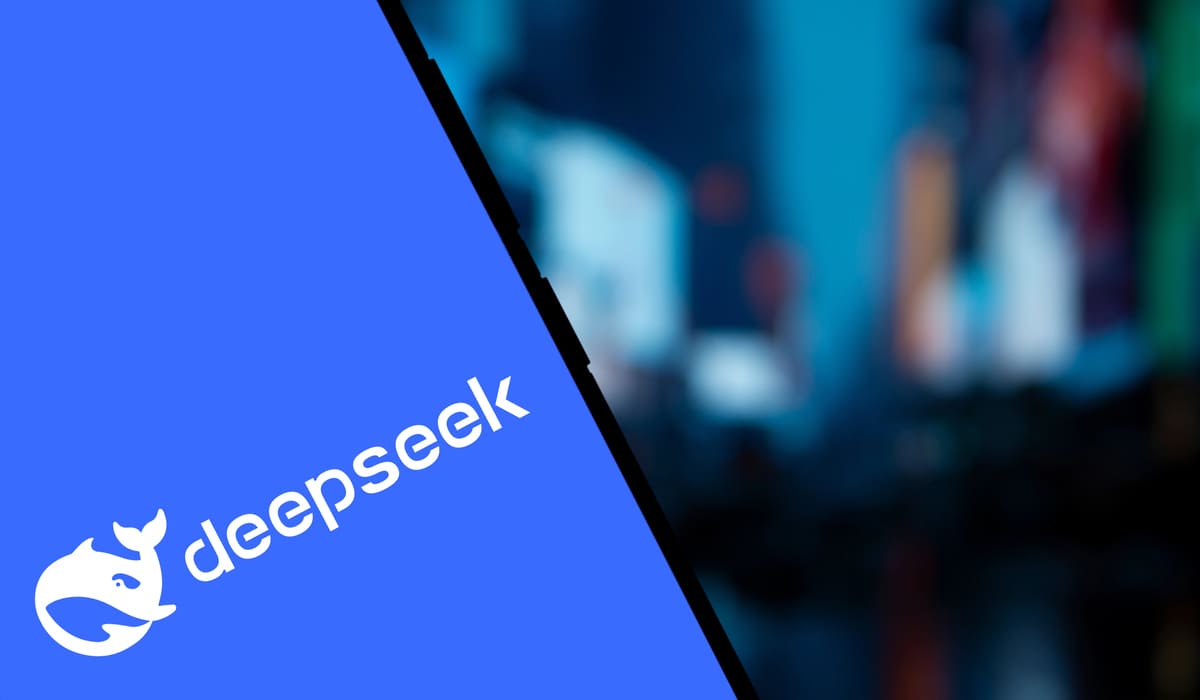
DeepSeek’s energy Consumption is shaking up the AI industry, raising questions about the massive investments Western companies are making in energy-intensive infrastructure, such as the U.S.-backed $500 billion Stargate project. Could this innovation redefine the future of AI?
DeepSeek’s Disruptive Impact
DeepSeek has sent shockwaves through stock markets, data center energy forecasts, and even Western Big Tech strategies. The burning question: Is it really possible to train and deploy a chatbot at a fraction of the cost and energy consumption of OpenAI, Meta, or Microsoft? What are the actual energy demands of DeepSeek, the AI startup founded by Liang Wenfeng in 2023?
Microsoft CEO Satya Nadella called DeepSeek’s latest chatbot model “impressive.” OpenAI’s Sam Altman used the same word. This comes just as OpenAI, alongside Oracle and SoftBank, announced the Stargate initiative at the White House: a $500 billion investment in AI infrastructure to solidify U.S. dominance in artificial intelligence.
DeepSeek’s Energy Consumption: 50-75% Less Than Competitors
Compared to the exorbitant costs of AI development in the U.S., DeepSeek’s efficiency is staggering. The company reports that training its v3 model—the predecessor of the latest R1—cost just $5.576 million. By contrast, Meta’s AI training costs for 2024 are projected at $65 billion, while Microsoft’s investment is expected to reach $80 billion.
Since training costs are directly linked to energy consumption, this efficiency has profound implications. According to DeepSeek’s own research papers—pending third-party validation—their servers consume 50% to 75% less energy than Nvidia’s latest GPU units. This reduction is especially crucial for data centers, which require vast amounts of electricity to power AI models.
H800 vs. H100: The Energy Efficiency Factor
DeepSeek’s energy efficiency could significantly impact the tech industry’s power demands. AI infrastructure in the U.S. largely relies on Nvidia’s high-energy-consuming H100 GPUs. For context, a cluster of 100,000 H100 chips requires approximately 150 MW of power.
DeepSeek, however, operates using Nvidia’s H800 chips—an alternative imposed by U.S. export restrictions, as H100 chips cannot be sold to China. The H800 chips are more power-efficient, and an equivalent AI cluster running on them would consume just 75 MW—almost half the power demand of H100-based systems. This efficiency could drastically cut data center energy consumption, reducing both environmental impact and operational costs.
Just 2,000 Chips for DeepSeek-V3
How has DeepSeek achieved such efficiency? When the company launched its V3 model right after Christmas, it immediately proved capable of competing with leading chatbots from OpenAI and Google. But the real breakthrough wasn’t just in performance—it was in resource optimization.
DeepSeek’s research team disclosed that they used significantly fewer chips than their competitors to train their model. While major AI companies rely on supercomputers with 16,000+ chips, DeepSeek achieved comparable results using just 2,000. This strategic approach could mark a turning point in AI energy efficiency and resource allocation.
The Future of AI Infrastructure
As the AI race accelerates, DeepSeek’s success raises fundamental questions about energy efficiency and investment strategies. If a Chinese startup can train competitive AI models using fewer resources, will Western tech giants need to rethink their approach? And will projects like the $500 billion Stargate initiative still be justified in an industry moving toward leaner, more sustainable AI development?
DeepSeek’s impact is only beginning to unfold, but one thing is clear: energy efficiency is now a key battleground in the AI arms race.